Case Studies
Demonstration Of Machine Learning For Sea Asset Identification
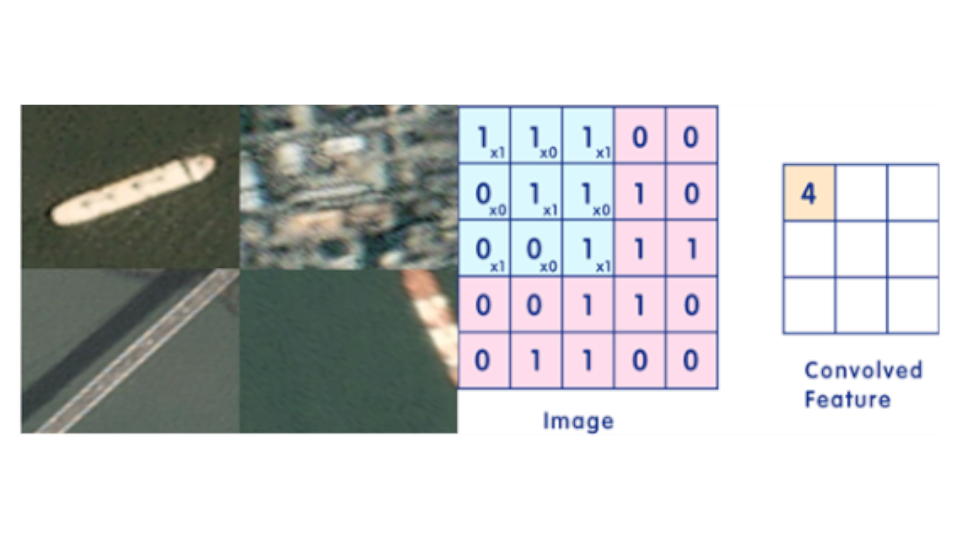
Target Identification refers to the ability to detect characteristics like thermal signature, shape edges and motion to distinguish target from the background. Data, feature, decision, or information fusion is necessary for high confidence target classification to be achieved using multiple sensors and sensor modalities. The architecture of target identification can be divided into three functional blocks: i) data acquisition ii) signal processing iii) target recognition and tracking. The task is to systematically analyse, locate and label a particular target among non-targets in a scene.
Target recognition and tracking is done using feature extraction and selection: the extraction of a set of numbers which characterize regions of data. It then processes the features for decision making (classification). Classification of individual pixels is the primary task to which several approaches exist. Targets are represented by little number of pixels in SAR/ISAR images. Target features are calculated with the help of few methods such as statistical classification models, image processing techniques (SAR/ISAR, photographic), neural networks and multisource data integration.
An image database contains images of multiple objects where sensors perceive images from same or different angles. Testing is done using the created database as input data. The parameters that could be changed in the testing are neural networks input layer size, size of training and testing. The reflected signal in visual sensors carry much information about the target which can be utilized for solving the target identification problem.